Fintech and Generative AI Juggernaut
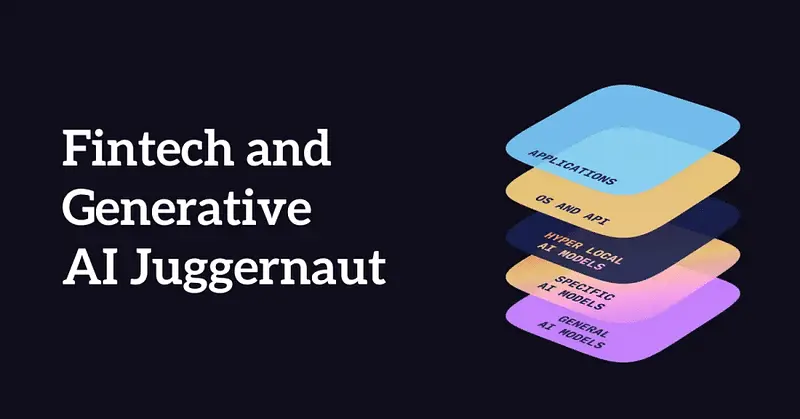
Get Easy & Instant Credit Now
Nurture your financial health with Potlee
You might have wondered why financial services haven't been targeted by Generative AI companies: well, GAI gives 90% accuracy, and financial services demand 100%. The limited data-sharing ability caused by regulations and compliance requirements poses a challenge in training models. But it would be folly for GAI to write off fintech and vice versa. GAI can be a critical ingredient in delivering financial services and making humans 10X more productive. Fintech has many use cases which are suitable for GAI.
Till late 2021, fintech attracted 20% of total VC funding globally. Then it faced an unexpected fall from grace in 2022 with regulatory bans, failing unit economics and rising interest rates. Publicly traded fintech companies' valuations fell rapidly. The global fintech funding fell 46% compared to 2021, according to CB insights. So what has taken the top spot for VC investing now? It is generative AI.
Where is all the money going in generative Al ?
Distribution of generative Al funding, 2021 - 2022
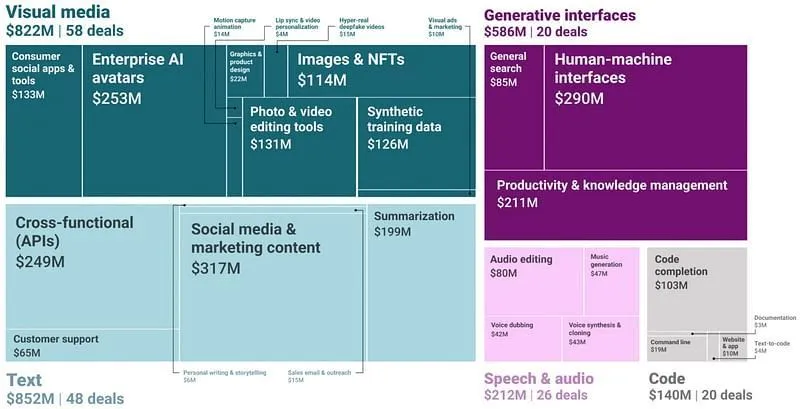
Source: CB Insights
Why so much noise around Generative AI ?
Machine learning has been better than humans at perceiving things and optimizing tasks. But now, thanks to new GAI models, they have broken the cognition barrier. GAI can also understand and interpret data and information in a meaningful way. This has led to an explosion in technology. And now, we can all experiment with it thanks to free tools like DALL-E and Chat GPT from Open AI, which have quickly captured our attention and sparked our imagination.
Eerie silence around GAI for Financial services
Financial industries, by design, are conservative and not early adopters of any technology. Financial companies demand 100% accuracy, and currently, GAI is amazing and powerful, but it's anything but accurate or precise. There is no room for error in this sector as a single bad decision can result in heavy losses, paying hefty fines, regulatory bans, tighter scrutiny or can even cost the license to operate. 100% accuracy is required in specific use cases like underwriting, regulatory checks (AML, KYC, BSA/AML, OFAC screening), and accounting/financial reporting.
Another reason is financial data is sensitive data which is available on the need to know basis, and the compliances are so strong that even internal teams cannot share their data with another team if not deemed fit by compliance. So how will these companies train the model if data can't be shared?
Instances where AI failed to achieve expected result resulting in bad publicity, criticism and revenue revision.
Global Instances:

Indian Instances:

Yet GAI for fintech is an idea whose time is yet to come, but just not now. Let's see this in the next section.
How GAI is adopted in fintech won't be the same as in the first-generation apps?
The role of AI in the end product varies quite a bit for still emerging applications created using foundation model APIs. Foundational LLM models rely on publicly available internet data and are adept at performing general tasks without extra training. Nonetheless, specialized tasks require specialized training data, workflows, or product wrappers to enhance AI performance. Overall, all these apps share three fundamental components.
- The foundation model that can be accessed through an API
- Fine-tuning the model with specialized or proprietary data including human interaction with the model so it improves over time
- Finally, a product or workflow that can be designed to make the best use of the AI's output
The variability of the role of AI in these apps is determined by the level of value provided by components (2) and (3). At one end of the spectrum, referred to as GaaS or Generative-AI-as-a-Service, there is a thin layer of (2) and (3). In contrast, at the other end of the spectrum, referred to as GaaC or Generative-AI-as-a-Component, there is a thick layer of (2) and/or (3)

For example, Jasper uses GPT-3 on the GaaS side to create content for B2B and B2C marketing and sales purposes. GAI is utilized to generate copy automatically, which is usually better than copy produced today. On the other hand, Notion has launched Notion AI, a feature that embeds GPT-3 as a "digital assistant' into its workflows, making GAI a component of its offering that improves product quality. Notion sits on the GaaC side of the spectrum.
As GaaS companies continue to mature, we expect they will build more and more IP around their core to differentiate, creating the ability to use their own data for a specific purpose effectively. The top ones will successfully evolve into GaaC companies (and thrive). In other words, as this market continues to mature, we're likely to see more and more GaaC companies.
We believe GAI apps that sell to financial services companies are likely to be on the GaaC side of the spectrum, where AI is delivered as a component in the broader software or workflow process. Two primary reasons for that are financial services data is not part of the publicly available internet datasets to train LLM, and secondly, companies in this market will not buy GAI to do a job till it's not 100% accurate.
What will it take to unlock the next level of innovation in financial services?
Despite the challenges associated with using GAI in fintech, we believe that this technology has enormous potential to transform financial services. Rather than using generative AI as a service (GAAS), it can be used as a component (generative AI as a component, GAAC).
In simple terms, fintech companies can offer generative AI as a component of their offering rather than using it just to enhance a process. There can be multiple ways of using generative AI as a component. Let's take a look at possible applications for any financial product. All of them can be classified under below three categories.

Distribution: How financial institutions sell their services as a product
- Customized marketing: This can help in increasing cross-selling by generating customer-specific messages to market new products
- Customized pricing and fee optimization: Customized pricing is based on the customers' creditworthiness and their financial behavior
- Personalized investment portfolios: GAI can create customized customer portfolios by analyzing historical market data, customer financial data, etc
- Improved client onboarding and automation of document management: AI will streamline client onboarding, automate KYC verification, enhance compliance with regulations, and improve security
Manufacturing: How the financial institutions create their product
- Fraud Detection: GAI system will enable real-time monitoring of banking transactions and algorithms to detect deceptive patterns more accurately
- Prediction of creditworthiness based on alternate data: GAI can eliminate biases in decision-making by analyzing alternate data to determine creditworthiness, such as payment history and financial behavior, instead of relying solely on credit scores
- A predictive model to estimate non-performing asset (NPA): GAI can predict potential NPAs by analyzing available data, enabling lenders to make provisions in their balance sheets
- Financial Planning and Analysis (FP&A): Enhance the FP&A team's forecasting accuracy by analyzing internal data such as revenue, cost, profit, and external data like interest rates and economic indicators
- Product Selection: GAI can simplify financial products for customers, increasing the chances of a sale by helping them understand their options, compare products
Servicing: How financial institutions deliver their product to customers
- Chatbots: GAI-powered chatbots provide 24/7 customer support, handle financial queries, provide advice, and assist with the company's account management
- Customer relationship management: GAI-powered customer relationship management (CRM) systems enable businesses to manage customer interactions and provide personalized experiences
Although GAI can produce amazing results, it's not a simple ‘ready-set-go’ technology. It is still in its nascent stage and requires a cautious, methodical approach to the introduction in the financial sector. Before going live with just a fundamental model, companies need to train the model for all possible scenarios and determine the accuracy of the prediction done by GAI, so they can know where human intervention is required rather than blindly following the model.
Businesses must prioritize identifying areas where GAI can provide the most value rather than simply chasing the AI dream. Failure to do so could result in wasted resources and missed opportunities for growth and success.
Another way to think about it, if today’s generative AI can get to 90% accuracy and a human review can get to 100%, you are making a human 10X more productive and hitting the accuracy threshold required to deliver financial services.
Get Easy & Instant Credit Now
Nurture your financial health with Potlee